Monitoring of frailty and dependence with wearables and machine learning
M. J. Rodríguez-Fórtiz, F. García-Moreno, M. Bermúdez-Edo, J. M. Pérez-Mármol, J. L. Garrido
Full text PDF 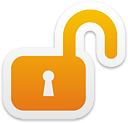
( Download count: 208)
AbstractInformation and Communication Technologies are increasingly used to maintain a healthy aging, both for monitoring and intervention. Currently we are working in this line, contributing with a software system to collect and analyze health data from the elderly and their environment in a holistic (multi-sense, combining different sources of information) and ecological (while the users carry out their activities of daily living) way (García-Moreno et al, 2019) (Figure 1). We have developed a system with a microservice architecture, which self-adapts to the devices used to collect the data and the kind of analysis applied. Currently we are focusing on frailty and dependence, with the goal to prevent them, monitoring physical health, cognitive status, mental health (emotions) and social relationships. To perform holistic and ecological monitoring, we use wearables (e.g. writs, watches and headbands), and smartphones, which sensors also can collect environmental and physiological data from users. We federated sensory data with other health data and analyzed them to monitor the elderly and to detect anomalies; trying to prevent illnesses and dangerous situations. We use artificial intelligence techniques to analyze the data collected over time. With these techniques we identify patterns, classify individuals, and forecast health status. In particular, we have detected dependence, and frailty or pre-frailty status (associated for example with weight loss, depression or sadness, and social isolation). The early detection of these pathologies can trigger an intervention program in health to reverse these problems.Keywords: frailty, dependence, services architecture, machine learning techniques
M. J. Rodríguez-Fórtiz, F. García-Moreno, M. Bermúdez-Edo, J. M. Pérez-Mármol, J. L. Garrido (2022). Monitoring of frailty and dependence with wearables and machine learning. Gerontechnology, 21(s),1-1
https://doi.org/10.4017/gt.2022.21.s.509.opp3