Self-learning AI method for anomaly detection in ambient assisted living environments
D. Gibietz, D. Helmer, E. Godehardt, H. Hinkelmann, T. Hollstein
Full text PDF 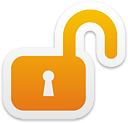
( Download count: 112)
AbstractThe demographic shift in Germany, along with the resulting rise in the average age of the population and the growing shortage of skilled workers in geriatric care, present challenges that demand innovative solutions. The aging population is accompanied by a growing number of individuals requiring care (Statistisches Bundesamt, n.d.). Elderly people, who live alone, are at risk of all kinds of accidents going unnoticed, leaving them without help for hours or even days. This can result in a decline in health and, in severe cases, even death. Advancing digitalization offers opportunities to support older people in maintaining their independence and living at home, improving their quality of life and reducing the workload of family members and caregivers. By using a wide range of sensors, it is already possible to recognize gradual changes in a multisensory manner at an early stage. Time-based observations of the duration of activities or everyday routines over time can provide initial indications of decreasing or unusual activities. For example, increased activity at unusual times can be a sign of sundowning syndrome and therefore dementia (Rindlisbacher & Hopkins, 1992). To achieve this, a self-learning system is being researched that adapt to individual users and continuously learn their normal behavior. This will enable early detection of changes and enable preventative measures to avoid accidents and maintain health. Movement patterns and habits vary from person to person. Therefore, an approach is needed that goes beyond a pre-trained, generalized system and takes into account and continuously learns specific individual patterns.Keywords: anomaly detection, ambient assisted living, machine learning, data fusion, deep learning
D. Gibietz, D. Helmer, E. Godehardt, H. Hinkelmann, T. Hollstein (2024). Self-learning AI method for anomaly detection in ambient assisted living environments. Gerontechnology, 23(2), 1-1
https://doi.org/10.4017/gt.2024.23.s.921.opp