Application of machine learning and numerical analysis to classify tremor in patients affected with essential tremor or Parkinson's disease
N.D. Darnall, C.K. Donovan, H-Y. Tseng, P. Barthelmess, D.C. Lin, S. Aktar, P.R. Cohen
Full text PDF 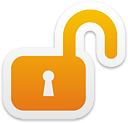
( Download count: 2146)
AbstractThe overall goal of this study was to compare the accuracy of various data analysis techniques to quantify tremor severity (TS) in a clinical context, with the aim of improving the reliability (context consistency and inter-rater agreement) of tremor evaluation in patients with Parkinsons disease (PD) or essential tremor (ET). Ten patients with either PD or ET were asked to perform several tasks used in the clinical practice for the characterization of tremor. Three-axis gyroscopes in a Shimmer device measured angular velocities of the wrist of each subject for postural, kinetic, spiral tracing, and resting scenarios, and a digital pen recorded subjects tracings of an Archimedes spiral printed on paper. Gyroscope data were used for training and testing a supervised machine learning algorithm to classify TS and for root mean squared (RMS) numerical rating of TS, while digital pen data were analyzed numerically to quantify tracing deviations from the spiral and obtain a tremor rating. We evaluated the performance of our proposed methods compared to clinicians diagnostic rating. The machine learning method matched the clinical rating with 82% accuracy, the digital pen with 78% accuracy, and RMS with 42% accuracy. We obtained the best accuracy of 82% using the decision tree machine learning approach with gyroscope data measured with the Shimmer.Keywords: tremor rating; spiral trace; digital pen; gyroscope; machine learning
N.D. Darnall, C.K. Donovan, H-Y. Tseng, P. Barthelmess, D.C. Lin, S. Aktar, P.R. Cohen (2012). Application of machine learning and numerical analysis to classify tremor in patients affected with essential tremor or Parkinson's disease. Gerontechnology, 10(4), 208-219
https://doi.org/10.4017/gt.2012.10.4.002.00